Simplify for Success - Conversation with Anjana Susarla
- Santoshi M
- Jul 14, 2021
- 19 min read
Updated: Aug 10, 2023
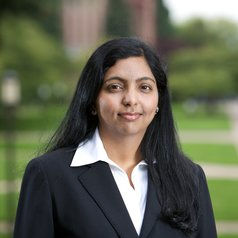
Anjana Susarla was on #SimplifyforSuccess, a podcast series presented by Meru Data and hosted by Priya Keshav to discuss IG programs.
As a professor of Responsible AI, Anjana spoke about AI-based bias and discrimination and why organizations should build and deploy AI in a responsible manner.
She discussed how data bias, bias from the method of training, and decision bias create a biased AI tool, and steps that can help build more responsible AI.
Listen to the podcast here:
Transcript:
Priya Keshav:
Hello everyone, welcome to our podcast around simplifying for success. Simplification requires discipline and clarity of thought. This is not often easy in today's rapid paced work environment.
We have invited a few colleagues in data and information governance space to share their strategies and approaches for simplification.
Today we will be talking with Anjana Susarla. Anjana earned an undergraduate degree in mechanical engineering from the Indian Institute of Technology, Chennai, a graduate degree in Business administration from the Indian Institute of Management, Calcutta, and PhD in Information Systems from University of Texas at Austin. Her research interests include the economics of information systems, social media analytics and the economics of artificial intelligence.
Her work has appeared in several academic journals and peer-reviewed conferences such as the Academy of Management Conference, Information Systems Research, International Conference and Information Systems Management Science and MIS quarterly.
Anjana Susarla has been the recipient of William S Livingston Award for outstanding graduate students at the University of Texas, a Steven Schrader best paper finalist at the Academy of Management and Association of Information System’s Best Publication Award. She has worked in consulting and experiential projects with several companies. She has been interviewed in and had op-eds research quoted and published in several media outlets such as Associated Press, BBC, Fox News, Houston Chronicle, Huffington Post, National Public Radio, NBC Washington Post, the week, wired World Economic Forum and Yahoo Finance.
Today, Anjana and I will be talking about responsible AI.
Anjana Susarla:
First of all, what do we mean by responsible AI? The use of artificial intelligence should not lead to discriminatory impacts on people?
Likewise, we you know Europe has recently introduced a whole bunch of regulations and they also talk about, at some point you need human intervention.
So what is that point? We need some sort of boundaries or guardrails around this kind of deploying of AI. Similarly, we talk, we won't worry about privacy. We also worry about all the societal or environmental consequences of AI and some accountability. So these are all different dimensions of what we call responsible AI and I think some realization is common into industry, especially things like credit and also automated hiring. I would say there's just so much of AI used in hiring that we have to understand if it's leading to some kind of discriminatory impacts, or you know, are we treating all the different groups in the population equally and so forth.
Priya Keshav:
How do you even build like that awareness of everything we touch has some sort of AI component to it, and understanding what is that AI behind it understanding the decisions that are being made in a legitimate fashion if they are being legitimately done and then you have data-related risks, right? Like the input and you know, output that comes in. But then you also have the security related risks, like what if it gets tampered? Whether I'm a corporate decision maker or a regulator, right? So how do you even get your arms around some of these risks. So how, in your mind, would you approach it?
Anjana Susarla:
I would approach it, I think ,by dividing it into five different boxes. There maybe some overlap, but we first start by understanding the data environment.
Where is our data even coming from? And maybe we need to consider some sort of de-biasing metrics for where the data is coming where we are building our models.
And that brings us into some of these contentious issues like facial recognition for instance.
So, if you are getting those kinds of data, are we putting some guardrails around that process? Second, we have to look at the business context and what are some rules that our business operates in and what are some regulatory guidelines there ? If you're building models using lots of data , there are many protected attributes, right? For example, gender-based discrimination is not legal and so forth, but there can be proxy discrimination.
So, what if instead you're building some AI-based model which takes a variable which is very correlated with gender, then what happens?
So, we have to do some 2nd order testing to establish those kinds of issues, right? The third one is, and I think this is the thing that we still haven't done much as in the business world and that is we need some compliance and auditing. So, if we need audit, then you know the business world is familiar with something like Sarbanes Oxley compliance. Do we have a similar algorithmic accountability? Who is going to do the auditing? Do we have independent third-party auditors? Do we have rules or do we have decision points where we can do that-and that would be the third dimension
Fourth dimension is just essentially understanding for post decision analysis, understanding how the model builds itself. What goes into the AI, and, I think, finally we need some explainability, right? So this is like, in my opinion, we need some kind of design framework that essentially should have some sort of questions and checkpoints for organizations, ensuring that AI is built in responsible manner and we can mitigate some bias.
I mean there may not exist anything like completely biased-free I, but do you use any fairness tools, like IBM’s360 fairness tool? And there's another tool called “Aequitas”. So, I mean there are some of these tools that have been developed out there.
But do we have some practice, and do we have maturity in deploying those tools ? So similarly, you know, we talk about process maturity et cetera and software engineering. At some point we need to be more mature about how we are building and deploying AI in the organizations.
Priya Keshav:
Yeah, I absolutely agree with that. I think some of the issues with software engineering will apply to AI as well, right?
You talked about design, so when it comes to design, the right models are being used. Obviously, the right data was used to train it and then the third part is, you know, as it's being deployed, how do you know that it's learning the right way? And then how do you know it's secure? Like in this and nobody has altered the data that is going in or the model itself. So, you know, right now I don't think there is a lot of diligence or accountability, or any kind of audit framework in place at all.
Probably if it is, it's a very small, subset of the population is thinking about it. But to build this at a massive scale where AI is ubiquitous and it is part of every software that you have developed, it's not a very easy task unless it's well thought out.
Anjana Susarla:
Yes, and I think you absolutely nailed it. The scalability is the issue, you know. Think of a company like Facebook. I think now it's valued at about 1 trillion, right? Think of a content moderation problem, which is also a responsible AI challenge, the more broader problem than what some organizations would face. How are you going to do content moderation at scale? Think of the massive amount of content that people are creating on Facebook on a daily basis on so many different countries in different languages.
And you know, still, there have been tremendous advances, and I think we have to use AI to spot the problems also created by AI in in a certain way right by looking for misinformation or you know, flagging things that look questionable. I think the two things will be, one is the scalability of these approaches-if you think of large banks and the volume of data that they handle. The second issue is, you know, in the context of things like software engineering, you have very well established.
Starting with some of these initiatives that happened come in when people were building, let's say aircraft and some of that thinking processes migrated to the software engineering world.
Do we have a similar process by which you have enterprise architects who have grappled with these problems in different contexts, and they can bring some of that thinking? I think that that's one of the biggest challenges facing the business world today, right? Like we are using AI without thinking too much into what's going into these black box systems and at some point, we have to start questioning. Would that mean that we require regulations like in Europe-there's a lot of regulations happening that are jumpstarting this whole process in the United States, for all of us who are grappling with responsible AI challenges in the US, then that's an open question of, you know what's the chicken and egg problem?
Would we have some algorithmic accountability type of regulations or should companies just self-govern themselves and take up some responsible AI practices? I think that companies like Microsoft and IBM are trying to invest and raise awareness of these issues. So I think that's a good first step.
Priya Keshav:
Yeah, I mean obviously, you know Microsoft has even called out AI as a risk in their annual report, right?
So those are all good steps, but you know if you are, let's say, not a Microsoft but an average retail company or a manufacturing company, right?
The question is AI and all these risks that we're talking about? Because we always use examples of Facebook, Uber, so is it more a Facebook and Uber problem than for most of us who sort of don't deal or don't think that we deal with AI on a regular basis?
How much of our decision making is impacted by AI and what kind of reputation and ethical risks can be there?
Anjana Susarla:
I think the issue of you know, calling it algorithmic bias sometimes puts people off the hook because in my opinion anytime you are doing any kind of automated decision making could be using Excel spreadsheet. But there's still some models and assumption, I think the the people who talk about AI and biases in AI have, I think, done a very good.
I think one thing they've done a great job is in highlighting some of these challenges. For example, Amazon. of course, this is a very large tech company. They built a resume screening type of tool and automated tool that can happen in any company, right? But one thing that happened was the historical data in the tech industry disproportionately happened to be men who were recruited.
So when you're using some of these methods from self-learning algorithms or anything. Essentially, what the algorithm learned to do is to prioritize male resumes over female resumes. So, ultimately, Amazon scrapped the tool right? So this problem itself, maybe it won't happen on the scale of Amazon but it can happen in any company. So, the question that responsible AI poses to any enterprise in highlighting what are some ingredients that go into your decision-making process.
It doesn't have to be fancy deep learning model. Essentially, any type of predictive methods predictive analytics that you're using, we'll still have to examine- should we consider desperate impact? Should we consider these things like equalized odds? What are certain now?
Criteria and making sure that all the across-gender, across-protected category, other that we are not treating different groups of people differently. Essentially, there's historically some biases and we are not repeating those biases because the historical training data, we're just using without de-biasing that data, right? I'll give one more example. I think there's this tool called Compass, which is used for a lot of judicial sentences. Now I think some researchers did this audit of compass system and what they found is essentially composes, they're looking at some 100 and something variables, but you could get actually more explanatory results with just very simple two to three variable model. So these are kind of examples, I'm just trying to illustrate my point, but the more we look into these use cases of AI bias, I think the two three things that are clear is bias, it does not have to be on a very large scale. It can happen in a small scale as well, and second, the biases in AI can be lots of times coming from data bias.
And the third is we need some way to audit and perform some kind of “what if” counterfactual scenarios. Google Research produced a tool called “What if”- all these would actually help companies do those kind of exposed checks.
Priya Keshav:
When it comes to bias in data, it might not just be about AI at all.
I mean AI just makes things in steroids. Like you said, it’s just analytics, yes can you start rolling out analytics program? How many times do you go back? You know you kind of say data speaks for itself and data can be manipulated.
We've seen that again and again, right? So it's all about how you, sort of slice and dice the data, and if you sort of give the wrong numbers, it comes up with the wrong conclusions and so it also applies to kind of doing that due diligence on any type of data-related analytics that you sort of do, whether it's with AI or without AI.
Anjana Susarla:
Yeah, this is absolutely what I feel as well. I think we are talking about AI as if the previous generation of technologies did not have these biases.
Actually, it's the opposite. I think it's that by using AI, we are able to see that biases exist better, right? So I think another example I want to share is from this UnitedHealth Group. They relied on some sort of decision making process. I wouldn't exactly call it AI, I mean it's automated. Maybe they were using spreadsheets as input to some program. There was a software program that was trying to prioritize who gets critical care when someone is admitted to the emergency.
So the group of researchers at various universities in the United States did this study that was published in a journal called Science, a very prestigious journal. What it found is algorithm is less likely to refer black people compared to white people who were equally sick to these programs that are meant to improve the quality of care. That is because how are they assigning risk scores? The risk scores were assigned to patients on the basis of the total health care costs that were accrued by that patient in the previous year.
Now, for everything else being equal, black patients were less likely to use the healthcare system, so the algorithm is considering the average black person to be healthier than the average white person.
So, is this a problem of AI? It is not in my opinion any issue, it is an issue of data.
And it's also an issue of the decision making process.
You know how the program was built, and fundamentally what it says is there are certain flawed assumptions about how people are using health care, and that's what any audit that we do is more also about the decision-making biases rather than, you know, just AI bias per say.
Again, as I said, the AI community has been at the forefront of highlighting some of these biases, but this can apply in any kind of decision making scenario.
Priya Keshav:
Yeah, so we talked a lot about data-based biases, right? But what about the models itself? I particularly look at or it could be even data, but model and data together. For example, what is very ubiquitous everywhere is essentially customer support, like chat boxes and customer support responses, right?
So you can completely ruin the reputation of a company if some samples into that model or the data, model-uses to respond to customers or price fixing like. There might be threshold limits, but again, what if a number of transactions happen where you know the prices are artificially manipulated?
You can have a lot of things like that. Earlier, it used to be more of either hybrid process or a manual process but now it's become completely automated where maybe there are threshold limits and some checks and balances. But what happens if some of those are tampered with or changed or altered?
Anjana Susarla:
Absolutely. I mean, you know there was a fantastic blog post on medium titled “How to create a racist AI without really trying”. So I think this data scientist Robyn Speer, what he did was if you're just using what is called sentiment scores for words which we use all the time in any analytics sentiment analysis.
But if you're taking sentiment scores for words and you're getting data from, let's say, you know, Yelp or any user-generated content like Amazon reviews, the algorithm is almost learning and teaching itself racist conclusions, and what's the reason for that? The reason is we are just, you know, the negative associations that people will say, “oh, this group of people are like this. Mexicans are like this. Chinese are like that.
Asians are like these. Indians are like that.” So we are just picking up those associations from just our method of training if we are putting sentiment scores. Does that mean we should not use sentiment scores? Absolutely not! There are methods where we can have these neutral labels. There's bias aware labeling so we could do word association analysis and see a de-biased, and you can still do the same. So, the chat bots and you know customer support is one of those areas where it's very important to pay some attention to the fact are we making any negative connotations? So, what's my method of how am I training my data? So we absolutely have to pay attention to those issues as well so you know, there are really three types of biases.
I think the bias can be data bias. It can be biased from the method of training and it can be decision biases.
And we can look at, you know, all three, like separately or together. It's not impossible to do those kind of audits or some performance tuning right to look at before debiasing and after debiasing.
Priya Keshav:
So if you are a person starting out like, let's say, you are a chief risk officer of a company and you were trying to think about at least financial services.
I think they're a little bit ahead in terms, so there's already been usually there are some algorithmIC audits that happen where people look at models and those kinds of things so. But if you are not from a financial services industryor even the technology industry, where do you start, how do you build a program to understand your risk and also kind of educate the rest of the company on the types of risks that can happen?
Anjana Susarla:
Yeah, that's a great question, so I think the awareness for that needs to come from essentially.
Can we sort of, you know, catalog these harms from automated decision making, right? You know, leaving aside the societal issues which can be employment, discrimination, insurance, education discrimination and so on.
The important thing is we should have some understanding of is there some economic loss to the people who are affected by this black box right now?
This question is sounding very broad, it's really because I don't see too many use cases or examples. We are still in the process, we are trying to build that vocabulary around risks. But, you know, I've been part of these working groups where we've looked at risks from, let's say automated lending risks from, automated recruitment and so forth. And so how do we know what are some key issues?
Are there some different groups of customers who are being treated differently? Is there some inconsistency in predicting how if the example can be from recidivism?
But if you know in judicial sentencing, one of the things that people want to predict is how likely is it that the person will commit the crime, again, right? And a lot of times the recidivism algorithms use ZIP code.
And so that creates a lot of biases right there for a chief risk officer in an enterprise. Similarly, we could be a little careful in sort of using these proxy discrimination, like ZIP codes or things like that.
So, if a tool is predicting success more accurately for men than women, that means it's not really identifying the best qualified women. Then we have all these fourth fifth rules. we can compare across different groups. We can also look at, you know, race and gender, sort of together. So, we have to look carefully at what data is collected and should we collect different data. What are some mitigation and should we do some oversight?
If there is oversight, what kind of oversight should you consider? And so these are all the different kinds of definitions.
But we also need to look at the organizational maturity. What are some key performance indicators and can we tie key performance indicators to these responsible AI principles? And that's I think, how we can consider, for example, the chat bots and financial services. This is where was the user being misled by something- can we do an audit of that right? So can we have a team inside the organization research these chat bot risk and what safeguards should we need when we are putting chat bots? Is there like an oversight? At some point we bring the human into the loop.
Priya Keshav:
You're basically raising a number of issues to consider, but I also want to take a step back and play a little bit of a devil's advocate, right?
We've seen a lot of growth in AI, and AI has become pretty much a part of every product that is out there, so it's being deployed more and more. And we have how many such cases or issues that we have really seen so in that sense, yes, there are some instances like Amazon stopping to use AI for hiring, right?
But outside the scope of this, is there any real harm that is happening in it or is it more of a theoretical problem than a practical problem.
Anjana Susarla:
Yeah question then, you know what's a best practice, fairness definition? How do we score them?
How do we continually monitor these? Are there some common usage ? Are there some risk data that we should not use at all or if we're using the data, we understand the risk in using those kinds of data. Like in auto lending for instance, auto insurance or auto lending, there is some biased lecture against younger drivers, right? So I mean, how do we understand the sources of these risks?
I would think it's each of these examples will have to be really fine tuned to that industry and that context.
That's why I said we need some organizational maturity of understanding for how many years has this company been designing and implementing these AI systems.
And that, I think, is what will guide the responsible use of AI. Just the maturity in understanding what they are doing.
Priya Keshav:
So in other words, you're basically saying that without awareness there's no way to kind of build a program or even understand if you're violating something or not.
Everything goes for a toss, like things fall apart. At that point it's too late.
Anjana Susarla:
Yes, yes, I totally agree with what you said. I think that a majority will come from within the organization, if they have a culture or where they can encourage people to raise some questions around what they are doing.
Because without that maturity, you won't be able to ask all these important questions, right? And we've seen some companies in the financial services industry. For example, there are some companies that have taken the lead in trying to bring some responsible AI within their organization and that leadership is, you know, the person who is the change agent within the company. Or maybe several change agents.
I think that's really the key to this kind of a transformation process. Without those individuals in the organization who understand the data, who understand the domain, and who understand the stakeholders and the big issues, we cannot just talk about AI responsible AI in some vacuum.
Priya Keshav:
Makes sense.
So let's talk a little bit about privacy and AI. So how do you think privacy can be impacted because of AI and what are some of the issues that you foresee from a privacy standpoint?
Anjana Susarla:
I think the challenge with privacy is even bigger, in my opinion, than just a responsibility within an organization. And the reason for that is each of us, I think, maybe most consumers I would say don't really understand how much data they have, for instance, companies like Google and all the online commerce world they have so much of data about us.
So, to some extent, I think when we talk about privacy and responsibility, it is very important for the AI system to be secure and respect privacy.
But when we go online, we post reviews, we are on social media and we're generating lot of digital traces about our lives. And companies have all that information.
And it's like, whether it's political campaigns or whether it's targeted ads, there's a whole lot of information that's out there about each of us. And companies are collecting and gathering and using all their data.
So I think the burden for privacy cannot be just on individual companies. It's a two-sided contract in my opinion, right? Like we are also, all of us are agreeing to forgo some privacy when we are part of this online ecosystem. So I think that's an important part of the conversation.
Priya Keshav:
I was just reading an article today about scraping of data from LinkedIn. Is that a breach or not? Somebody was arguing and it is actually an interesting point of view, right?
So I would kind of say yes. I'm posting some information on LinkedIn. While I agree with you that we post enormous amount of information online. And we need to be better aware of how that impacts our privacy, but sometimes there's a lot of information collected without us. For example, our Geo locations, right?
So it's not like you are actually consenting to that much information to be available online and to be publicly used and to be sold as records with a bunch of information.
So, I think AI to some extent, enables that process a little bit more, because now it can connect the dots a lot better than you know any other tool or human being could. So once you know somebody has trained an AI with that kind of data set, you could probably also pull information out of the models which kind of impacts privacy as well, right?
So, I while I agree with you, I also disagree with you so.
Anjana Susarla:
Well, I think when we talk about privacy.
And I mean not only privacy. I think they there are two different issues. One is privacy and other is data rights. We currently don't have a system in place where we can demand ownership of our data.
And again, that's something where ideally, I think you know the World Economic Forum and UN organizations have put out these principles of responsible AI, and that's also one of the data rights. I think giving individuals some control over data that's collected about them is very much part of those bigger picture initiatives, but how does that trickle down into corporate practice? That's still an ongoing debate in my opinion. I haven't seen much around that, I haven't seen much discussion around those issues is, I think right now at least.
Priya Keshav:
So any other closing thoughts on responsible AI?
Anjana Susarla:
Yeah, I think that we need more awareness about how AI affects our lives. Maybe we need high school students to have some sort of understanding, just like you have a civics lesson and so forth.
Like raising all these, you know, younger people to be sort of digital natives. We use so many apps, especially with the pandemic, so much of the learning has migrated online.
And some of these apps I've seen that in even my children’s classes they used to do this kind of differentiated curriculum in the classroom.
Now that happens through an app and it's like that even the teacher doesn't quite know how the app is assigning problems to a particular student, so there's just again so much of those black box decisions that affect our lives.
These things are happening in education and everything that we do almost and I think we just need more awareness of these issues.
Priya Keshav:
Yeah, that's the first starting point. With awareness comes understanding. With understanding comes the approach to managing some of this.
So what about frameworks? NIST is definitely trying to kind of provide some thoughts in how to manage responsible AI.
But in other frameworks that you're aware of.
Anjana Susarla:
Yeah, the World Economic Forum has some frameworks. There’s a working group, they have released a lot of guidelines. There's also the European Union, I forgot the name of that body, but the European Union has a similar to NIST, There are standards bodies, and those are actually very good. Some of their proposal, there was a leaked draft of some regulations, and those looked very good to me.
Priya Keshav:
Thanks Anjana, I've enjoyed talking to you.
Anjana Susarla:
Thank you.
*Views and opinions expressed by guests do not necessarily reflect the view of Meru Data.*
Comments