AI and Data Privacy in the Insurance Industry: What You Need to Know in 2024
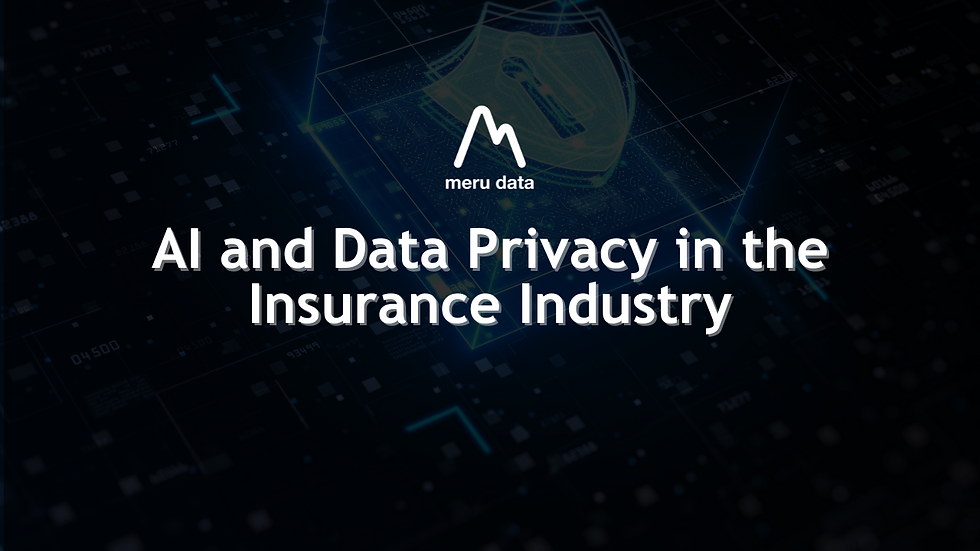
The insurance industry is no stranger to the requirements and challenges that come with data privacy and usage. By nature, those in insurance deal with large amounts of Personally Information (PI) which includes names, phone numbers, and Social Security numbers, financial information, health information, and so forth. This widespread use of multiple categories of PI by insurance companies demands that measures are taken to prioritize individuals’ privacy.
Further, in recent months, landmark cases of privacy violations and misuse of AI technology involving financial institutions have alarmed the insurance industry. While there is no doubt that embracing new technology like AI is a requirement to stay profitable and competitive going forward, let’s look at three main considerations for those in the insurance industry to keep in mind when it comes to data privacy: recent landmark cases, applicable regulations and AI governance.
Recent noteworthy cases of privacy violations and AI misuse
One important way to understand the actions of enforcement agencies and anticipate changes in the regulatory landscape is to look at other similar and noteworthy cases of enforcement. In recent months, we have seen two cases that stood out
First is the case of General Motors (G.M.), an American automotive manufacturing company. Investigations by journalist Kashmir Hill found that vehicles made by G.M. were collecting data of its customers without their knowledge and sharing it with data brokers like LexisNexis, a company that maintains a “Risk Solutions” division that caters to the auto insurance industry and keeps tabs on car accidents and tickets. The data collected and shared by G.M. included detailed driving habits of its customers that influenced their insurance premiums. When questioned, G.M. confirmed that certain information was shared with LexisNexis and other data brokers.
Another significant case is that of health insurance giant Cigna using computer algorithms to reject patient claims en masse. Investigations found that the algorithm spent an average of merely 1.2 seconds on each review, rejecting over 300,000 payment claims in just 2 months in 2023. A class-action lawsuit was filed in federal court in Sacramento, California.
Applicable Regulations and Guidelines
The Gramm-Leach-Bliley Act (GLBA) is a U.S. federal regulation focused on reforming and modernizing the financial services industry. One of the key objectives of this law is consumer protection. It requires financial institutions offering consumers loan services, financial or investment advice, and/or insurance, to fully explain their information-sharing practices to their customers. Such institutions must develop and give notice of their privacy policies to their own customers at least annually.
The ‘Financial Privacy Rule’ is a requirement of this law that financial institutions must give customers and consumers the right to opt-out and not allow a financial institution to share their information with non-affiliated third parties prior to sharing it. Further, financial institutions are required to develop and maintain appropriate data security measures.
This law also prohibits pretexting, which is the act of tricking or manipulating an individual into providing non-public information. Under this law, a person may not obtain or attempt to obtain customer information about another person by making a false or fictitious statement or representation to an officer or employee. The GLBA also prohibits a person from knowingly using forged, counterfeit, or fraudulently obtained documents to obtain consumer information.
National Association of Insurance Commissioners (NAIC) Model Bulletin: Use of Artificial Intelligence Systems by Insurers
The NAIC adopted a bulletin in December of last year as an initial regulatory effort to understand and gain insight into the technology. It outlines guidelines that include governance, risk management and internal controls, and controls regarding the acquisition and/or use of third-party AI systems and data. According to the bulletin, insurers are required to develop and maintain a written program for the responsible use of AI systems. Currently, 7 states have adopted these guidelines; Alaska, Connecticut, New Hampshire, Illinois, Vermont, Nevada, and Rhode Island, while others are expected to follow suit.
What is important to note, is that the NAIC outlined the use of AI by insurers in their Strategic Priorities for 2024, which include adopting the Model Bulletin, proposing a framework for monitoring third-party data and predictive models, and completing the development of the Cybersecurity Event Response Plan and enhancing consumer data privacy through the Privacy Protections Working Group.
State privacy laws and financial institutions
In the U.S., there are over 15 individual state privacy laws. Some have only recently been introduced, some go into effect in the next two years, and others like the California Consumer Privacy Act (CCPA) and Virginia Consumer Data Protection Act (VCDPA) are already in effect. Here is where some confusion exists. Most state privacy regulations such as Virginia, Connecticut, Utah, Tennessee, Montana, Florida, Texas, Iowa, and Indiana provide entity exemptions to financial institutions. This means that as regulated entities, these businesses fall outside the scope of these state laws. In other words, if entities are regulated by the GLBA then they are exempt from the above-mentioned state regulations.
Some states, like California and Oregon, have data-level exemptions for consumer financial data regulated by the GLBA. For example, under the CCPA, Personal Information (PI) not subject to the GLBA would fall under the scope of the CCPA. Further, under the CCPA, financial institutions are not exempt from its privacy right-of-action concerning data breaches.
As for the Oregon Consumer Privacy Act (OCPA), only 'financial institutions,' as defined under §706.008 of the Oregon Revised Statutes (ORS), are subject to a full exemption. This definition of a ‘financial institution’ is narrower than that defined by the GLBA. This means that consumer information collected, sold and processed in compliance with the GLBA may still not be exempt under the OCPA. We can expect other states with upcoming privacy laws to have their own takes on how financial institutions’ data is regulated.
AI and Insurance
Developments with Artificial Intelligence (AI) technology has been a game changer for the insurance industry. Generative AI can ingest vast amounts of information and determine the contextual relationship between words and data points. With AI, insurers can automate insurance claims and enhance fraud detection, both of which would require the use of PI by AI models. Undoubtedly, the integration of AI has multiple benefits including enabling precise predictions, handling customer interactions, and increasing accuracy and speed overall. In fact, a recent report by KPMG found that Insurance CEOs are actively utilizing AI technology to modernize their organizations, increase efficiency, and streamline their processes. This not only includes claims and fraud detection, but also general business uses such as HR, hiring, marketing, and sales. Each likely use different models with their own types of data and PI.
However, the insurance industry’s understanding of Generative AI related risk is still in its infancy. And according to Aon's Global Risk Management Survey, AI is likely to become a top 20 risk in the next three years. In fact, according to Sandeep Dani, Senior Risk Management Leader at KPMG, Canada, “The Chief Risk Officer now has one of the toughest roles, if not THE toughest role, in an insurance organization”
In the race to maximise the benefits of AI, consumers’ data privacy cannot take a backseat, especially when it comes to PI and sensitive information. As of 2024, there is no federal AI law in the U.S., and we are only starting to see statewide AI regulations like with the Colorado AI Act and the Utah AI Policy Act. Waiting around for regulations is not an effective approach. Instead, proactive AI governance measures can act as a key competitive differentiator for companies, especially in an industry like insurance where consumer trust is a key component.
Here are some things to keep in mind when integrating AI:
Transparency is key: Consumers need to have visibility over their data being used by AI models, including what AI models are being used, how these models use their data, and the purposes for doing so. Especially in the case of insurance, where the outcome of AI models has serious implications, consumers need to be kept in the loop about their data.
Taking Inventory: To ensure accuracy and quality of outputs, it is important to take inventory of and understand the AI systems, the training data sources, the nature of the training data, inputs and outputs, and the other components in play to gain an understanding of the potential threats and risks.
Performing Risk Assessments: Different laws consider different activities as high-risk. For example, biometric identification and surveillance is considered high-risk under the EU AI Act but not under the NIST AI Risk Management Framework. As new AI laws are introduced in the U.S., we can expect the risk-based approach to be adopted by many. Here, it becomes important to understand the jurisdiction, and the kind of data in question, then categorize and rank risks accordingly.
Regular audits and monitoring: Internal reviews will have to be maintained to monitor and evaluate the AI systems for errors, issues, and biases in the pre-deployment stage. Regular AI audits will also need to be conducted to check for accuracy, robustness, fairness, and compliance. Additionally, post-deployment audits and assessments are just as important to ensure that the systems are functioning as required. Regular monitoring of risks and biases is important to identify emerging risks or those that may have been missed previously. It is beneficial to assign responsibility to team members to overlook risk management efforts.
Conclusion
People care about their data and their privacy, and for insurance consumers and customers, trust is paramount. Explainability is the term commonly used when describing what an AI usage goal or expected output is meant to be. Fostering explainability when governing AI helps stakeholders make informed decisions while protecting privacy, confidentiality and security. Consumers and customers need to trust the data collection and sharing practices and the AI systems involved. That requires transparency so they may understand those practices, how their data gets used, the AI systems, and how those systems reach their decisions.
About Us
Meru Data designs, implements, and maintains data strategy across several industries, based on their specific requirements. Our combination of best-inclass data mapping and automated reporting technology, along with decades of expertise around best practices in training, data management, AI governance, and law gives Meru Data the unique advantage of being able to help insurance organizations secure, manage, and monetize their data while preserving customer trust and regulatory compliance.
Comments